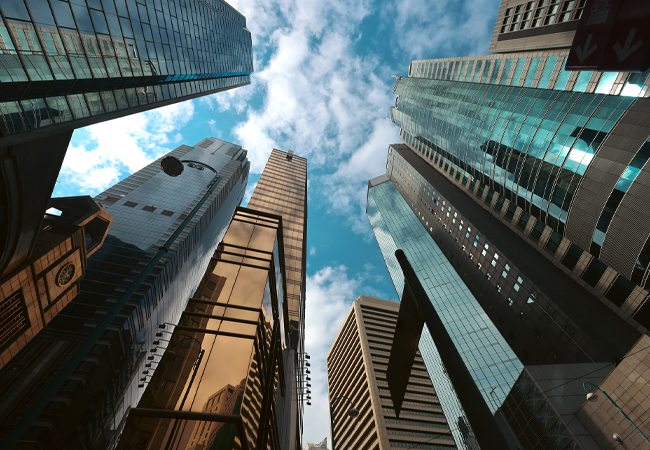
Lessons from the pilot projects can be adopted across Hong Kong
A major Hong Kong property developer is set to slash carbon emissions across its estate using artificial intelligence (AI) and big-data analysis, after two successful pilot projects resulted in huge energy savings and a payback of only two years.
The properties of MTR Corporation Limited (MTR) encompass 16 shopping malls, four office buildings, and more than 110,000 residential units across 57 property estates. To explore the potential of cutting carbon and greenhouse gas emissions from its estate, MTR implemented two pilot projects.
The first involved the use of a cloud-based data analytics software platform to optimise the central chiller plant at Two International Finance Centre (Two ifc), a commercial development in Hong Kong’s Central District. The second looked at developing an integrated AI solution to enhance energy efficiency and customer experience at the Elements MTR Mall in West Kowloon. Both initiatives resulted in significant annual energy savings and improved building performance.
Big-data analysis at Two ifc
Built in 2003, Two ifc is a skyscraper and integrated commercial development on the waterfront of Hong Kong’s Central District. Rising to a height of 415 metres, it is the second-highest structure in Hong Kong and boasts a total floor space of about two million square feet.
The pilot project involved integrating a cloud-based, big-data analytics software platform to optimise chiller plant. (Big data is described as large and diverse datasets that cannot be processed easily using traditional data-processing techniques). The initial key performance indicator (KPI) was a minimum 5% annual energy saving at the central chiller plant.
The Two ifc chilled-water system encompassed a seawater plant, circulation pumps, and chilled-water and seawater distribution networks, with a total installed plant capacity of approximately 9,800 tonnes
of refrigeration (TR).
The cloud-based software platform securely collects and analyses data from ultrasonic temperature and water flow transducers, energy meters, and existing building management and energy management systems (BMS/EMS).
Real-time data is analysed to generate actionable insights aimed at enhancing energy efficiency. The platform has a secure connection to offsite storage provided by the software service provider. It incorporates fault detection and diagnostics (FDD) software that analyses hard-wired and virtual data points using time-series data. This allows for effective comparison, diagnosis, evaluation and reporting of the chilled-water system’s performance.
The digital team
For the Elements and Two ifc projects, internal MTR teams work with system maintenance contractors, and technical and digital consultants.
Their responsibilities include conducting energy management assessments, identifying operational enhancements, refining logic based on operational data, and overseeing ongoing commissioning.
They also review the current physical and digital infrastructure, and establish workflows and methodologies for data processing and integrating with existing systems.
The data-processing and analysis results undergo regular reviews and assessments to gauge effectiveness and system performance.
This continuous evaluation ensures that energy saving and operational improvement measures remain optimised and aligned with desired outcomes.
The project team prepares monthly reports to review data analysis, insights and energy savings, allowing for continuous adjustments to the system settings. A key strategy involves optimising the operational sequence of 10 seawater-cooled chillers based on their coefficient of performance (COP), ensuring that the most efficient chiller operates first.
In terms of energy reduction, the project team maintains rigorous measurement and verification standards to comply with international and local regulations.
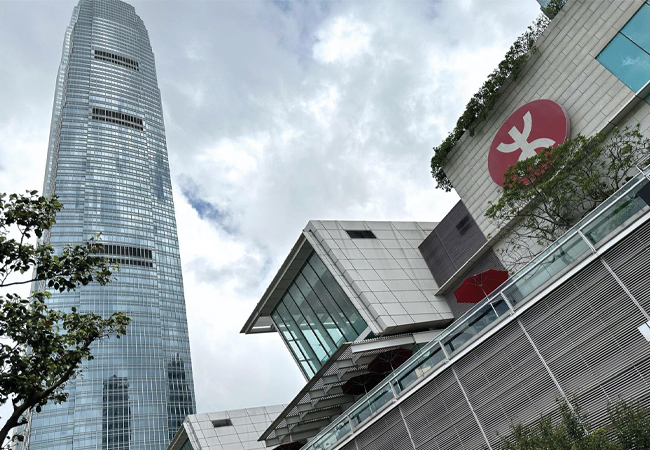
AI measures at the Two ifc commercial development cut energy use by 9% in 2023
Historical operation data from the BMS serves as a control set for comparison before and after implementing the cloud-based platform. The system continually records trend logs and monitors performance against the energy-saving opportunities, allowing for timely adjustments to optimise energy savings.
AI optimisation at Two ifc
Energy-saving measures that were implemented include:
- Aligning cooling load demand with the chiller’s highest COP to maximise cooling output while minimising power consumption
- Optimising startup and shutdown times to reduce energy waste before occupancy hours
- Shifting peak cooling demands to maintain power consumption within limits
- Using stochastic and generalised additive models to forecast cooling load and adjust chiller settings accordingly
- Using FDD rules and AI models to fine-tune operational parameters of chiller equipment to ensure it stays in a predefined energy efficient range (the zero energy band).
Elements MTR Mall
Opened in 2007, the Elements MTR Mall has a retail area exceeding one million square feet and accommodates more than 120 stores.
Elements undertook a proof-of-concept project aimed at developing an integrated AI solution to enhance energy efficiency and improve customer experience. The initial KPI was a minimum 3% annual energy saving at the central chiller plant.
Like Two ifc, the chilled-water system at Elements included a seawater plant, seawater and chilled-water circulation pumps, and chilled-water and seawater distribution networks. It had a total installed plant capacity of around 7,000TR.
The development of an air conditioning and mechanical ventilation optimisation model uses advanced machine learning techniques to address the dynamic characteristics of HVAC systems and fluctuating environmental conditions.
Supervised learning, where algorithms learn patterns and relationships between inputs and outputs, plays a crucial role in understanding complex, non-linear correlations.
Additionally, self-adaptive reinforcement learning, which involves dynamically adjusting the learning process based on feedback, means the system optimises equipment performance based on real-time energy usage and COP.
This continuous learning process enhances the model’s accuracy over time, driving energy efficiency. The AI model forecasts cooling load every 30 minutes, using historical data, weather forecasts, and solar radiation.
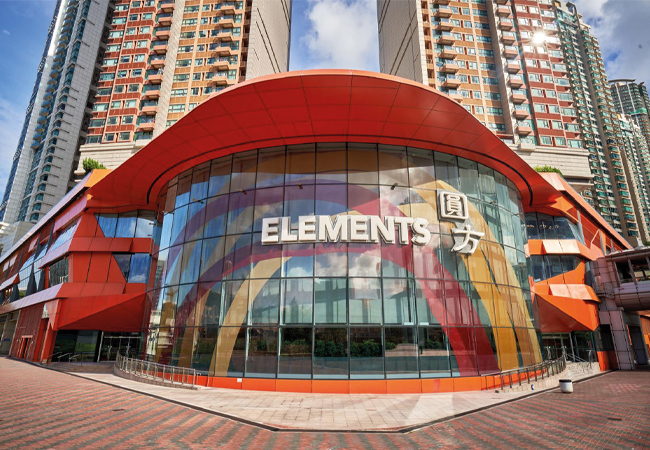
Big-data analytics cut annual energy use by 9% at the Elements MTR shopping mall
Insights from the model guide equipment combinations and chilled-water setpoints, allowing chiller plants to proactively manage cooling demand fluctuations and optimise energy usage. The model is improved through iterative testing and operator feedback.
Real-time data collection is vital for optimising the system based on actual demand patterns. Integrated people-counting techniques use CCTV to analyse foot traffic in different mall zones, which identifies peak usage periods.
This data informs energy-saving strategies, such as adjusting air handling unit supply temperatures while maintaining thermal comfort. More than 45 indoor air quality (IAQ) sensors monitor parameters such as CO2 levels, temperature and humidity, enabling the AI to refine recommendations for fresh air optimisation. Compliance with local and international air quality standards, including the Reset Air Standard for airborne viral transmission, is maintained, with studies indicating excellent IAQ ratings, even during peak periods.
A data quality (DQ) assessment framework was developed to enhance AI model training. This framework identifies DQ issues and provides recommendations for the BMS. Following the assessment, a digital infrastructure upgrade plan was implemented to improve sensor accuracy and data completeness, which are essential for effective energy management analysis.
The optimisation model includes a visualisation platform that recommends optimal chiller configurations based on historical performance. A daily recommendation dashboard and a rule engine for real-time anomaly detection enhance predictive maintenance capabilities.
Setting up AI to optimise building performance
CIBSE technical editor Tim Dwyer on how to set up an AI model to optimise an environmental control system:
1. Define objectives
Establish key goals – eg, energy efficiency, cost reduction, occupant comfort, indoor air quality.
2. Data collection
Gather data from sensors and control systems such as indoor/outdoor temperatures, humidity, CO2 concentrations, occupancy, energy consumption, weather conditions, historical performance.
3. Preprocessing the data
Clean and prepare the data by handling missing or inconsistent values. Perform feature engineering to extract meaningful inputs – for example, variables such as ‘average occupancy during working hours’.
4. Choose the AI model type
Select one to fit the system’s needs:
- Regression models: To predict system requirements such as heating or cooling demands based on current conditions
- Reinforcement learning: To learn optimal control policies through continuous system feedback
- Neural networks: To understand complex relationships between factors such as occupancy, weather and system performance.
5. Train the AI model
Use historical and real-time data to predict and optimise system operations HVAC systems.
6. Integrate with building systems
Connect the AI model with the BMS via IoT platforms or APIs, allowing real-time adjustments to be made.
7. Implement feedback loops
Where the AI model receives real-time data and dynamically adjusts environmental controls to optimise energy efficiency and comfort.
8. Test and validate
Perform simulations and pilot tests to evaluate how well the AI improves system efficiency and occupant comfort. Fine-tune the model to meet performance objectives.
9. Continuously monitor and update
Monitor the system and update the AI model using new data to ensure it adapts to changing conditions.
Results
The innovative pilot project enabled Two ifc to achieve a total energy saving of more than 2.3 million kWh of electricity over two years (January 2022 to December 2023), translating to a total reduction of more than 1,500 tonnes of CO2e. The energy savings percentages stood at approximately 11% in 2022 and 9% in 2023.
Meanwhile, at Elements, the AI optimisation system delivered an annual energy saving of approximately 9% during the period August 2023 to July 2024, amounting to more than 1.3 million kWh of electricity saved and a CO2e reduction of more than 715 tonnes.
The projects have already been recognised by the industry. They have garnered four building awards that acknowledge the project teams’ exceptional accomplishments in designing and operating energy-efficient structures.
About the author
Ethan Poon is the assistant chief project and maintenance manager at MTR Corporation